The future of Automated Logistics
Reasons for automated logistics
In an evermore competitive market, ecommerce phenomena such as unified commerce are driving automation in operations with the aim of minimising errors and maximising efficiency in warehouse processes.
The consolidation of ecommerce means that pallets are no longer the predominant unit load in logistics centres. This is illustrated by the 2020 Warehouse/DC Operations Survey, drawn up by Peerless Research Group for Logistics Management. This report finds that 70% of respondents dispatch their goods in combinations of pallets and boxes.
The complexity of controlling operations isn’t the only issue facing logistics professionals. Ecommerce has also slashed delivery times, forcing all supply chain links to adapt to new challenges such as returns management or increased costs due to last-mile delivery.
Against a backdrop of rising demand and higher logistics costs, automating operations such as goods flows, warehouse slotting and order processing can make the difference. Technology helps to ensure tight stock control and to guarantee efficient service, with deliveries in the time and place previously agreed upon with the customer.
Automated logistics encompasses RFID devices to drones for moving loads
What does the future of automated logistics hold?
It’s important to understand automated logistics as a comprehensive process involving the adoption of new technologies that are here to stay. This point is noted in a study by consulting firm McKinsey, which states that in less than a decade, AI will play a leading role in all logistics processes: “Many operations could be automated by 2030, as artificial intelligence takes over the many repetitive activities that logistics companies perform. We expect to see fully automated high-rack warehouses, with autonomous vehicles navigating the aisles. …Warehouse-management systems will keep track of inventory in real time.”
The data support this forecast. According to a study from MarketsandMarkets, between 2020 and 2026, the logistics automation market is expected to grow by 10% annually, reaching $88.9 billion.
The study Operations management of smart logistics: A literature review and future research, published by professors Bo Feng and Qiwen Ye in Frontiers of Engineering Management, lists the main trends that are transforming logistics. This article confirms that the logistics sector has rushed to implement smart information technologies such as RFID (radiofrequency identification) devices, blockchain, big data analysis, AI, and drones for visualisation, traceability and smart decision-making during the logistics phase.
WMSs play a key role in consolidating automated logistics in companies
The future lies not only in automating logistics operations performed inside and outside the warehouse, but also automating decision-making, eliminating any risk of error arising from manual management of products. In this scenario, digital systems such as a warehouse management system (WMS) or a manufacturing execution system (MES) take centre stage.
This commitment to technology has a primary objective: to maximise logistics resources to boost profit margins. The Supply Chain Leadership analysis from consulting company Deloitte points in that direction: 79% of businesses with optimised supply chains obtain turnover levels higher than the average for their sector.
Main automated logistics solutions
Automated logistics consists of the introduction of all types of technologies across the various supply chain phases: from production line supply to product storage and transport. These are some of the prime logistics automation solutions for each stage:
How to automate inventory control
A WMS is the ideal solution for ensuring rigorous control of all operations taking place in the warehouse, especially goods receipt and dispatch. This control provides the logistics manager with total product traceability, as well as real-time information on the available stock.
A warehouse management system acts as the brain of the facility, automating processes such as inventory control, turnover assignments for each item and slotting management based rules preset by the logistics manager.
How to automate storage, transport and order picking
Warehouse digitisation can be supplemented with storage solutions such as stacker cranes (AS/RS for pallets), which move the goods in the aisles and deposit them in the corresponding location. Another option is automated transport systems, e.g., pallet conveyor systems and automatic guided vehicles (AGVs), particularly when flows of goods are constant.
In the case of warehouses that don’t have fixed routes, automated logistics is supported by systems based on AI such as autonomous mobile robots. These machines are charged with moving unit loads such as pallets and boxes freely about the facility, as indicated by the WMS.
To aid the order picking process, one of the costliest and most complex phases to automate, companies deploy picking assistance devices e.g., pick-to-light, which optimise the tasks of the warehouse pickers. When order prep entails the handling of heavy loads or strenuous physical effort, pick & place robots can be installed. These are very useful for repetitive movements.
Automatic conveyors speed up the flow of goods
Automated logistics also includes digital solutions that enhance WMS functions to streamline goods routing outside the facility. These software programs efficiently organise the entire dispatch process to ensure that the goods are delivered to customers as quickly as possible.
But implementing digital systems isn’t the only way to go: there are also automatic solutions such as autonomous vehicles for moving loads in outdoor spaces. In addition, there are several systems on the market that use AI and whose widespread use is just around the corner. This is the case of drones, whose application will revolutionise certain processes, namely production line supply and ultra-fast deliveries (quick commerce).
Automated logistics: the road towards optimisation and efficiency
The replacement of manual operations with automated systems enhances all supply chain phases, from product transport and supply to management of locations in the warehouse.
Technologies such as automated storage systems, autonomous robots and drones complement the work of operators, reserving the work the employees do for operations that add value.
At 3S we manufacture solutions for logistics automaton that cover all our customers’ needs.
Looking to optimise resources in your warehouse and maximise productivity? Get a Free Audit today: one of our expert consultants will offer you the best solution to ensure the utmost efficiency in your logistics operations.
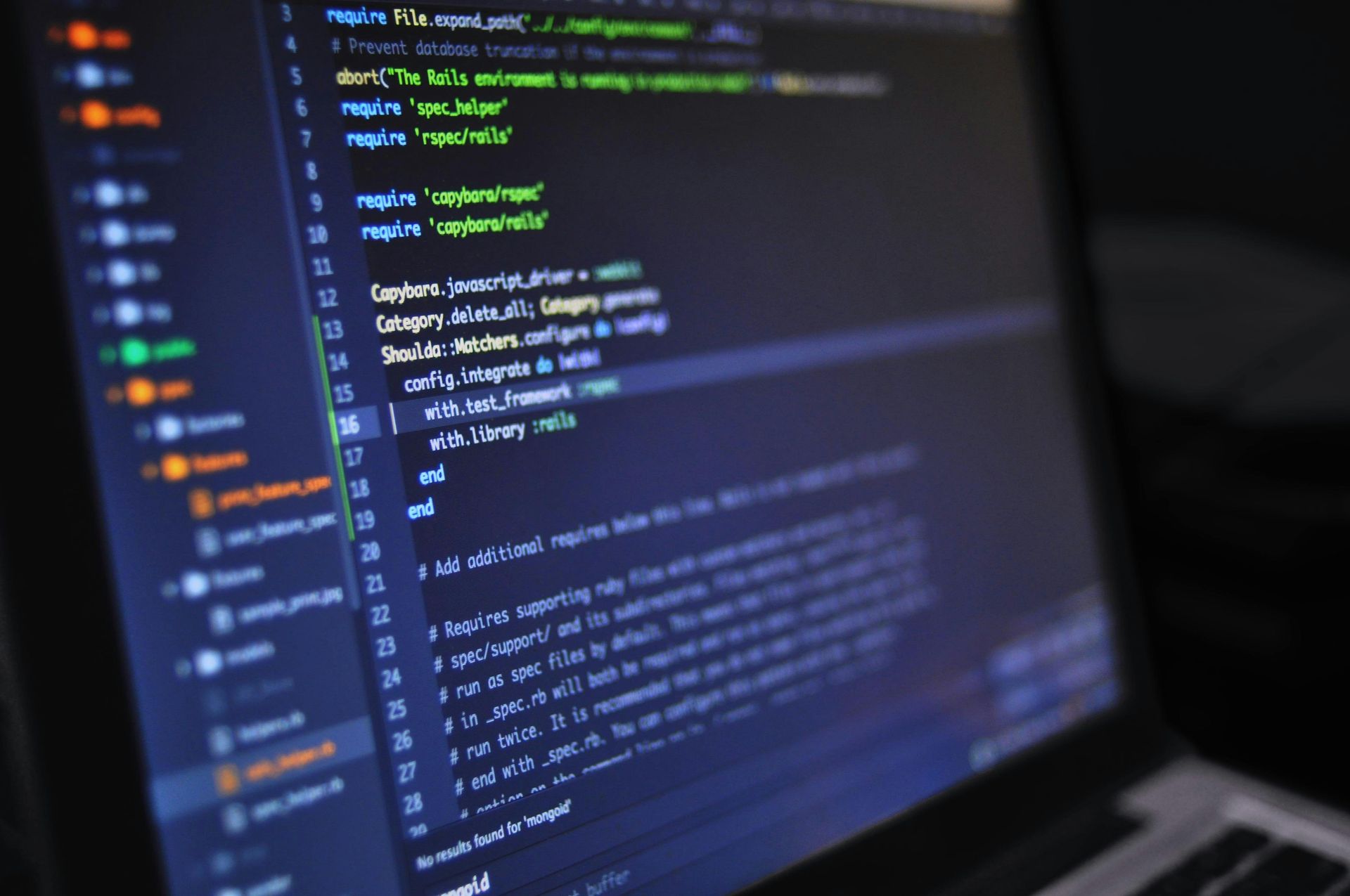
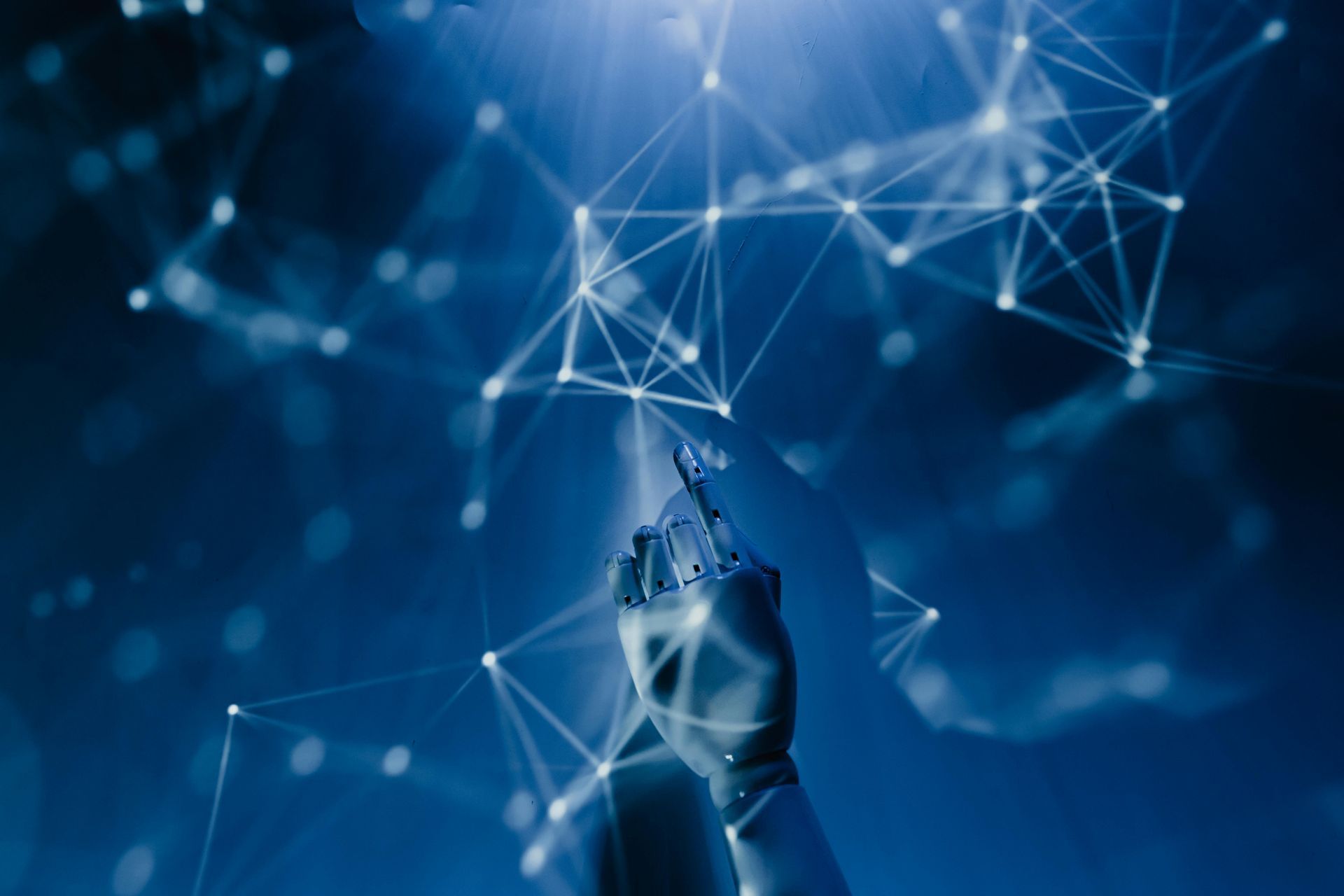